Files Included :
1 Introduction to Natural Language Processing (42.98 MB)
2 Regenesys Graduate Atributes (48.45 MB)
3 Course Contents - What You'll Learn (28.37 MB)
1 What is Word Embedding (121.16 MB)
2 Frequently Asked Questions about N-Grams (52.63 MB)
3 How Embedding Vectors Can be Created from Text (33.99 MB)
1 What are the Different Ways of Creating a Model for NLP Tasks (72.24 MB)
2 What kind of vectorization Tf Idf performed (55.22 MB)
3 Introduction to Recurrent Neural Network (45.76 MB)
4 Variations or Applications of Recurrent Neural Networks (RNN) (60.38 MB)
1 Introduction to Word Embedding and Word2Vec (61.43 MB)
2 Difference between CBOW and skip-gram Models (81.45 MB)
3 Doc2Vec and BERT Models Explained (48.67 MB)
4 Which Vectorization Method to Use (34.91 MB)
5 What are the Pre-trained Models and their Advantages & Limitations (27.72 MB)
6 Frequently Asked Questions about Pre-trained Models (76.44 MB)
1 What is Word Embeddings (46.42 MB)
2 Text Vectorization Using Word2Vec Model (62.33 MB)
3 Building CBOW and Skip Gram model (66.42 MB)
4 Getting Similar Words Using CBOW Model (118.01 MB)
5 Getting Similar Words Using Skip Gram Model (69.2 MB)
1 Token Based Text Embedding Trained on English Wikipedia Corpus (51.41 MB)
2 Using Tensorflow Hub Model (70.6 MB)
3 Feed Forward Neural Network Explained (101.74 MB)
4 Which Vectorization Method to Use (34.93 MB)
5 Example of Using Pre-Trained Models (72.41 MB)
6 More Questions About N-Grams (99.54 MB)
1 Overview of Text Summarization in NLP (71.89 MB)
2 What is NLP Text Summarization (31.74 MB)
3 Types of Text Summarization (105.42 MB)
4 Use CasesApplicationsEveryday Examples of Text Summarization (27.45 MB)
5 Steps for Extractive Text Summarization (44.92 MB)
1 Tokenization and Get Word Frequency (43.29 MB)
2 Getting Normalized Word Frequency and Sentence Tokenization (48.71 MB)
3 Calculating Score for Each Sentence and Ranking The Sentences (159.66 MB)
4 Creating a Text Summarization Application (41.71 MB)
5 A Gentle Introduction To Text Summarization by Jason Brownlee (36.27 MB)
6 How to Open Text Summarization Code File in Colab (54.37 MB)
1 How to import Spacy by using Pip Install Spacy (107.16 MB)
2 Removing Stopwords in Text Summarization (69 MB)
3 Getting Pre-Trained English Language Models from Spacy Library (64.07 MB)
4 Word Frequency Counter using NLTK (44.32 MB)
5 Normalizing of Word Frequency and Sentence Tokenization (32.11 MB)
6 Calculating Score for Each Sentence (46.44 MB)
7 Text Summarization using Sentence Scoring Method (182.75 MB)
8 Spacy English Model Memory Requirements (65.51 MB)
1 Getting YouTube Transcript Using YouTube Transcript api (133.32 MB)
2 Understanding Text Embedding with Word2Vec (61.65 MB)
1 Overview of Movie Recommendation System Using NLP (39.36 MB)
2 What is Recommendation System and its Advantages (47.35 MB)
3 Movie Recommendation Methods Using NLP (50.99 MB)
4 Introduction To Demographic Filtering (105.31 MB)
5 Introduction To Collaborative Filtering (66.67 MB)
1 What is Natural Language Processing(NLP) (45.03 MB)
2 History of Natural Language Processing (38.97 MB)
3 Why Natural Language Processing is important in Today's World (69.46 MB)
4 Applications of Natural Language Processing (36.5 MB)
5 Variations in Natural Language Processing Machine Learning (31.49 MB)
1 TMDB 5000 Movie Dataset (140.02 MB)
2 Uploading and Read in Some Fixed Folder on Your Drive (63.46 MB)
3 What Does df2 Contain (78.87 MB)
4 Introduction To Content Based Filtering (57.13 MB)
5 Computing Score for Every Movie using IMDB Weighted Rating Formula (163.73 MB)
6 Sorting Movies Based on Calculated Score (94.46 MB)
1 Finding Cosine Similarity Score With TF-IDF Vectorizer (102.47 MB)
2 Computing Cosine Similarity Matrix Using Linear Kernel (43.66 MB)
3 Getting the Pairwise Similarity Scores of All Movies (43.88 MB)
4 Sorting the Movies and Getting the Top 10 Most Similar Movies (53.5 MB)
5 Cast, Crew, Keywords and Features Used For Recommendations (197.11 MB)
6 Frequently Asked Questions About Movie Recommendation System (72.17 MB)
1 Averaging Word Embedding Vectors (100.83 MB)
2 Implementation of A Doc2Vec Model Using Gensim (91.28 MB)
1 Overview of Text Classification System (32.41 MB)
2 What is Text Classification System and Its Advantages (78.26 MB)
3 Step-by-step Explanation of Text Classification (194.47 MB)
4 Basic Text Classification in NLP (81.97 MB)
1 Installing Tensorflow Hub and Getting The Words Embedded (77.71 MB)
2 Defining LSTM Models (89.76 MB)
3 Encoding Code to Number Using Preprocessing (38.85 MB)
4 Building, Training & Testing the LSTM Model (65.45 MB)
5 Frequently Asked Questions About Using LSTM Models For Sentiment Analysis (152.46 MB)
1 Introduction to Sentiment Analysis Dataset (47.21 MB)
2 Counting Sentiment Value in Text Classification (79.2 MB)
3 Visualizing Sentiment Value Count (30.83 MB)
4 Downsampling The Dataset and Visualizing After Downsampling (22.49 MB)
5 Data Pre-Processing in Machine Learning Method (44.76 MB)
6 Visualizing Sentiment Analysis With Word Clouds (41.09 MB)
7 Naive Bayes Classifier in Machine Learning (85.92 MB)
8 TF-IDF for Sentiment Analysis (96.48 MB)
9 Frequently Asked Questions About Machine Learning Method for Sentiment Analysis (21.25 MB)
1 Sample Dataset for Natural Language Processing(NLP) Tasks (57.12 MB)
2 Natural Language Processing(NLP) Project - Core Steps for Success (90.99 MB)
3 Essential Python Libraries for NLP Projects (49.89 MB)
4 Mastering Regular Expressions (Re) Library (123.71 MB)
5 Regular Expressions (Re) Library for Data Cleaning (12.4 MB)
1 What is Tokenization in Natural Language Processing (NLP) (57.89 MB)
2 Differentiation between Stemming and Lemmatization (31 MB)
3 Data Cleaning Process in Natural Language Processing (36.2 MB)
4 Few Steps in Pre-processing for Natural Language Processing (NLP) (63.23 MB)
1 Understanding Text with Bag-of-Words (BOW) Model (119.26 MB)
2 Related Terms to Explore (123.39 MB)
3 Sample Example of Text Processing (45.67 MB)
4 Explaining N-grams in Natural Language Processing (NLP) (96.94 MB)
5 Applications of Language Models (29.31 MB)
1 Intro to NLTK for NLP with Python (67.99 MB)
2 Stemming and Lemmatization In Python (88.31 MB)
3 How to import and use stopwords list from NLTK (72.91 MB)
4 Tokenize text using NLTK in python (68.26 MB)
5 Removing stop words with NLTK in Python (84.92 MB)
1 N-Gram Language Modelling with NLTK (38.48 MB)
2 What are bigrams in NLP (110.41 MB)
3 How to Implement N-grams in Python (150.86 MB)
4 Using CountVectorizer for NLP feature extraction (146.69 MB)
5 Implementation of TF-IDF for NLP (63.98 MB)
1 Different Tokenization Methods in NLP (39.22 MB)
2 Stemming and Lemmatization Using nltk (64.23 MB)
3 Part of Speech(POS) Tagging with Stop words using NLTK (121.77 MB)
1 Python Regex match search methods (129.12 MB)
2 Substituting Patterns in Text Using Regex (76.43 MB)
3 Finding All Matches using findall Method (42.81 MB)
4 Finding All Email Addresses in the String (10.43 MB)]
Screenshot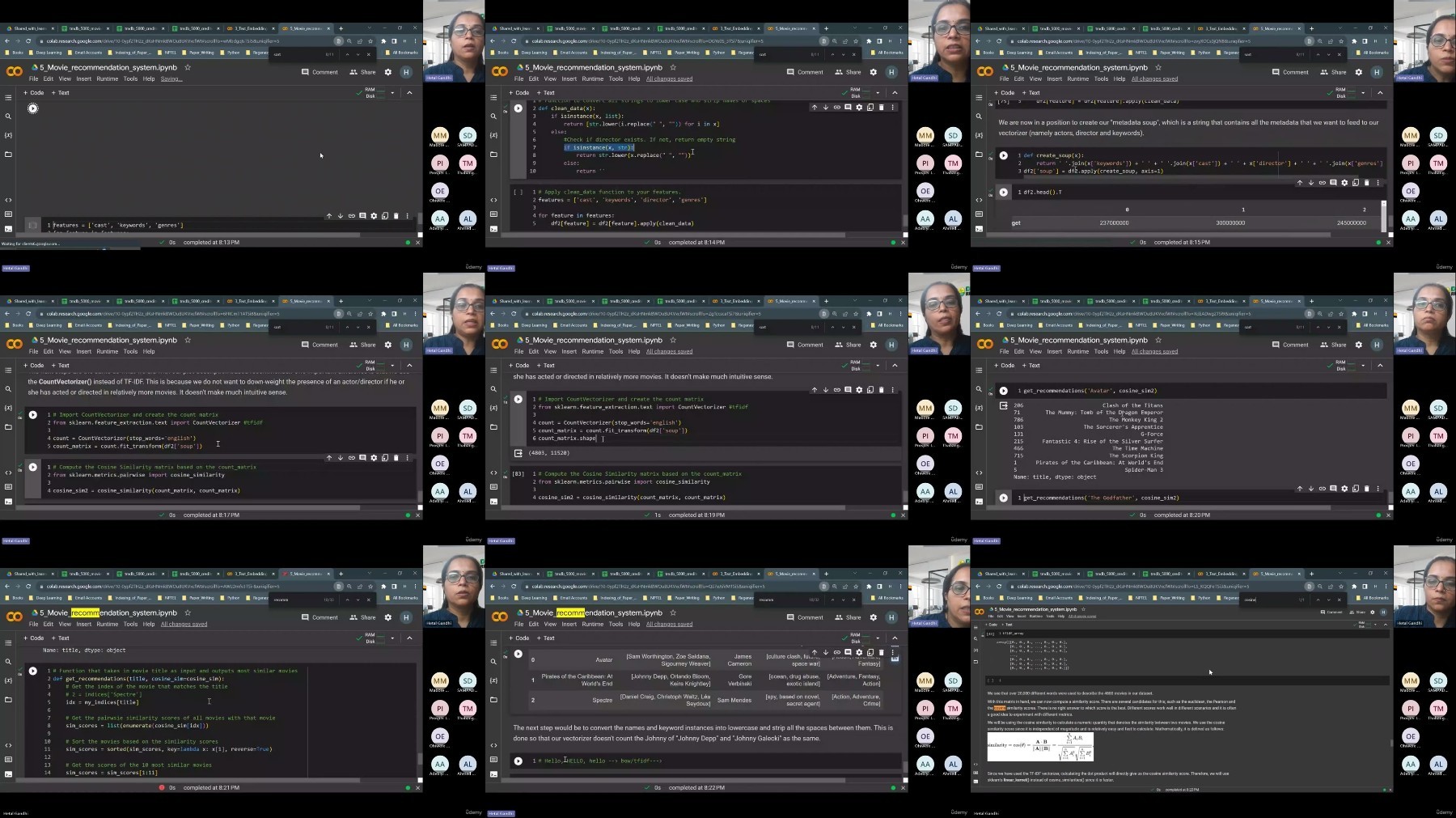